[Chem] Machine Learning-Assisted Exploration of a Versatile Polymer Platform with Charge Transfer-Dependent Full-Color Emission
Yinyin's collaborative work with the Leroux group uses machine learning to explore a new series of polymer dyes with emission covering entire chromaticity.
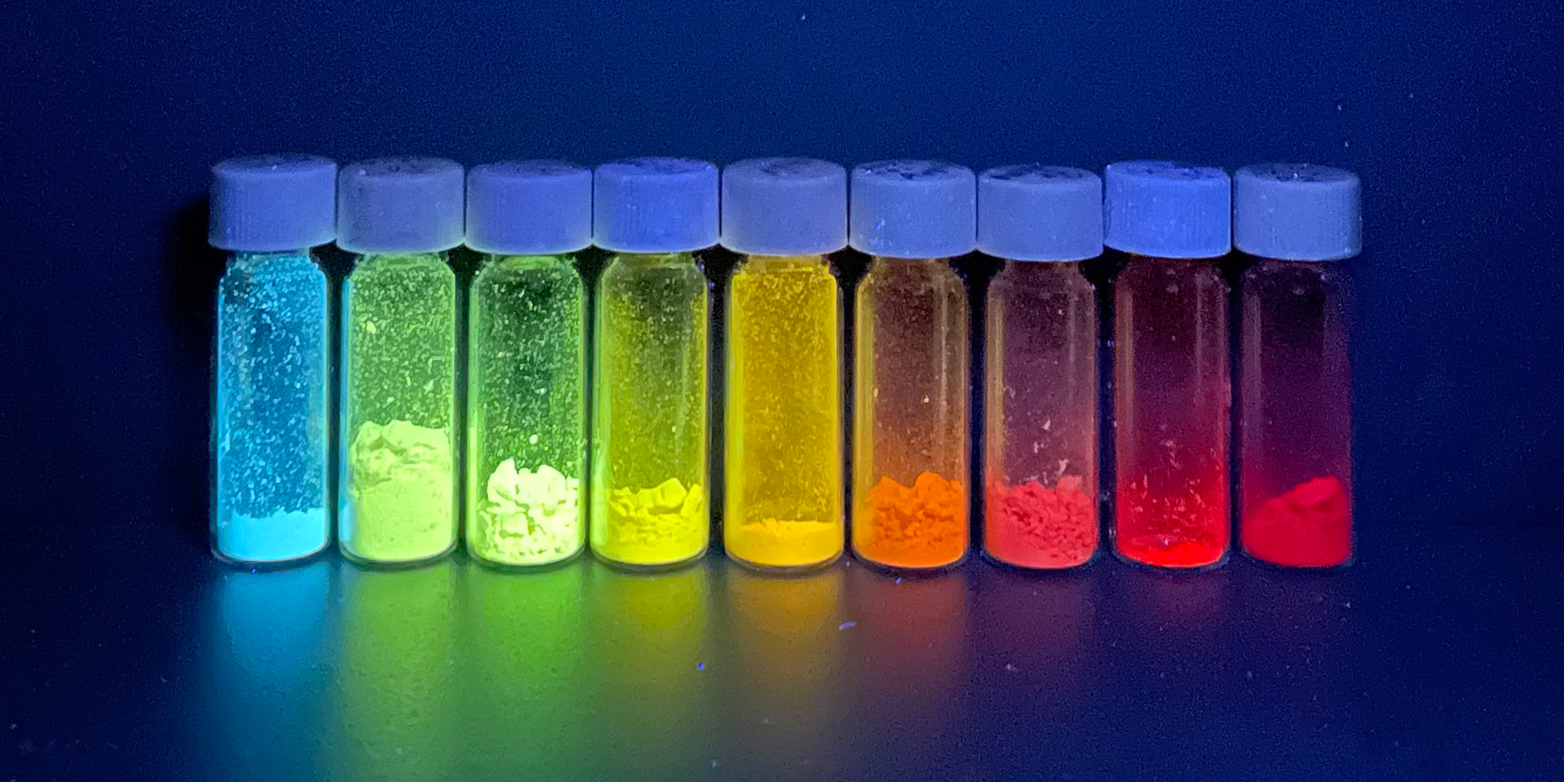
The development of color-tunable fluorescent materials with simple chemical compositions that are easy to synthesize is highly desirable but practically challenging. Here, we report a versatile yet simple platform based on through-space charge transfer (TSCT) polymers that has full-color-tunable emission and was developed with the aid of predictive machine learning models. Using a single-acceptor fluorophore as the initiator for atom transfer radical polymerization, a series of electron donor groups containing simple polycyclic aromatic moieties (e.g., pyrene) are introduced either by one-step copolymerization or by end-group functionalization of a pre-synthesized polymer. By manipulating donor-acceptor interactions via controlled polymer synthesis, continuous blue-to-red emission color tuning was easily achieved in solid polymers. Theoretical investigations confirm the structurally dependent TSCT-induced emission redshifts. We also exemplify how these TSCT polymers can be used as a general design platform for solid-state stimuli-responsive materials with high-contrast photochromic emission by applying them to proof-of-concept information encryption.